Research at IIIT-H
At IIIT-H, I work as Research Fellow at the HAI (Healthcare and Artificial Intelligence) vertical under the guidance of prof. Bapi S Raju (link). I enjoy designing computational models that aid clinicians with quick and accurate solutions. I have dedicated my time to two major tasks.
- Designing a self-supervised contrastive model to acquire histopathological whole slide images.
- Analyzing various orofacial representations for neurodegenerative diseases such as ALS (Amyotrophic Lateral Sclerosis) and Stroke
Histopathological Analysis
Technological advancements have aided clinicians by developing pathological tool kits for cancerous histological data. The visual examination of histopathology slides is one of the prime methods to inspect the prognosis of a tumor based on its morphological appearance. This process is time-consuming and needs a detailed evaluation from the high-resolution whole slide images (WSI's). Hence, we provide a decision support system using deep learning, which in turn analyses and interprets the tumorous regions from the WSI's. The existing literature focuses on cancers with high mortality rates, such as lung, colorectal, and liver. But, we instead focus on cancerous regions that are rare but have high mortality rates, such as the palate, small intestine, and meninges.
The existing deep learning algorithms provide explainable neural networks which locate the cancerous proportions in WSI's. But, the erstwhile literature does not provide valid justification regarding the representational structure of deep neural networks. As a result, we acquired a strategy, CKA, which would aid us in comprehending various internal aspects of neural networks.
It is well established in the literature that histopathological slide (diagnostic slide) images are one of the gold-standard techniques to analyze and assess cancerous regions and aid clinicians in predicting tumorous profiles. Specifically, developing technological tools can aid clinicians in analyzing the effectiveness of tumors. TCGA https://portal.gdc.cancer.gov/. study led to the establishment of massive archives of digital H & E WSI's of Carcinomas. These photos were captured at 20x and 40x magnifications and are nearly Gigapixels, which are visually challenging to examine and evaluate properly.
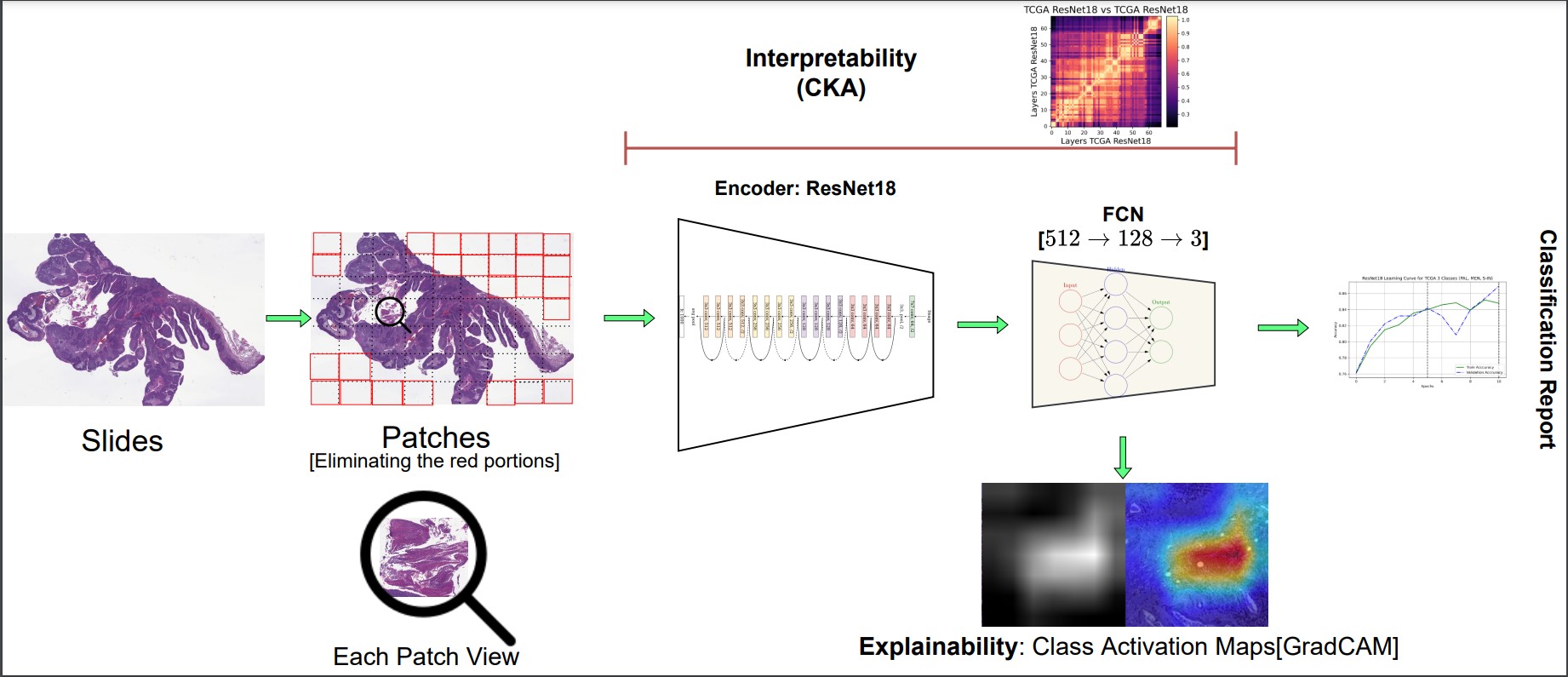
This work utilizes self-supervised (label-free) methods to extract representations and provide on-par performance with supervised approaches. This work is still in progress, and the publications and information regarding this work will be added soon.
Analyzing Orofcaial Distortions
Currently, neurodegenerative diseases are threatening with their severe implications and the perplexity in their diagnosis. Neurodegenerative diseases are very uncommon, and they are hard to comprehend and diagnose. Hence it is crucial to detect the specified variant of neurodegenerative disease and provide the appropriate remedy immediately. Some of these variants grow progressively, and others act momentarily, making the diagnosis challenging. Large publicly available datasets with facial videos, photos, and clinical information are necessary to improve the effectiveness of Face Recognition systems to encourage their application in clinical practice.
The role of Artificial Intelligence in clinical decision-making has played a crucial role in providing cost-efficient and robust throughput. Computer vision methods have significantly progressed in various Face Recognition systems. Hence, we pick a publicly available dataset, i.e., Toronto NeuroFace, which sheds light on developing unique and intelligent deep neural networks for automatically assessing motor speech problems and oro-facial deficits.
The details of the Toronto Neuroface data, i.e., subjected explicitly to the participants, data collection procedures, clinical evaluation of the videos, and underlying details of manual annotation of facial landmarks on a subset of frames are vividly illustrated in work https://slp.utoronto.ca/faculty/yana-yunusova/speech-production-lab/datasets/ This work is still in progress, and the publications and information regarding this work will be added soon.